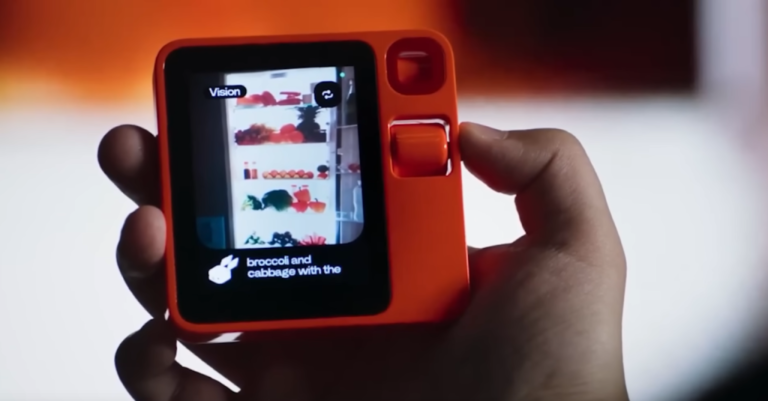
[ad_1]
💡 Definition: A Giant Motion Mannequin (LAM) is a sophisticated synthetic intelligence system designed to carry out human-like duties inside digital environments. Using neural networks and symbolic reasoning, it interprets and executes complicated instructions in purposes, like net navigation or on-line transactions, by instantly modeling and understanding the logic and construction of those interfaces.
Synthetic Intelligence (AI) has a brand new growth referred to as Giant Motion Fashions, or LAMs. These fashions are a sophisticated type of Giant Language Fashions (LLMs), which many people learn about. LLMs create textual content by guessing the subsequent phrase based mostly on enter.
LAMs go additional. They flip LLMs into ‘brokers,’ software program that may do duties alone. This implies they’ll do extra than simply reply questions; they’ll work in direction of a objective. This can be a large change as a result of it mixes LLM’s language abilities with the power to do duties and make choices on their very own.
LAMs are constructed to imitate the best way purposes and human actions work collectively. They use neuro-symbolic programming to do that with no need textual content. This can be a complicated space, and we don’t have full entry to those fashions but.
LLMs and LAMs are each AI fashions, however they’re completely different. LAMs can hook up with real-world methods, like IoT gadgets. This lets them do bodily duties, management gadgets, collect knowledge, and deal with info. They will automate complete processes, discuss to folks, adapt to altering conditions, and work with different LAMs.
LAMs are highly effective for a number of causes.
- First, they perceive complicated human objectives and switch them into actions.
- Second, they’ll neatly work together with the world, together with folks and altering conditions.
- Third, they join with real-world methods.
- Lastly, they flip generative AI from a easy instrument right into a useful associate in real-time duties.
LAMs have many potential makes use of. In healthcare, they may change affected person care with higher diagnostics and coverings. In finance, they may assist with threat evaluation, discovering fraud, and making algorithm-based transactions. Within the automotive business, they may enhance self-driving automobiles and security methods.
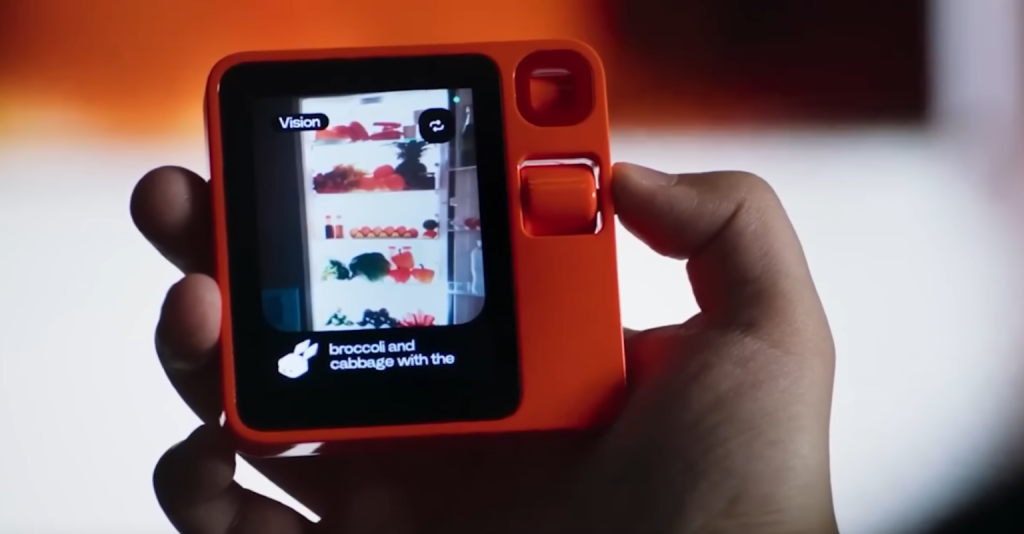
👉 Rabbit R1 Machine – What’s the Hype?
🐇 Software: A sensible instance of LAMs is the Rabbit r1 system, promoting for $199. It’s a small system, about half the scale of an iPhone, with a touchscreen and a rotating digital camera. It has a scroll wheel for straightforward navigation. The Rabbit r1 makes use of Rabbit’s AI working system (OS) and LAM. This lets it perceive and mimic human actions on know-how interfaces. Rabbit’s development exhibits how on-line interactions can grow to be simpler with no need apps.
LAMs are set to vastly influence AI’s future. They flip language fashions into ‘brokers’ that may do duties, making AI a real-time motion associate. Merchandise like Rabbit are already utilizing LAMs, opening up many new potentialities. This marks a significant shift in AI growth.
For instance, the concept of LLM-based working methods has been proposed not too long ago by Karpathy, Tesla’s former head of AI:
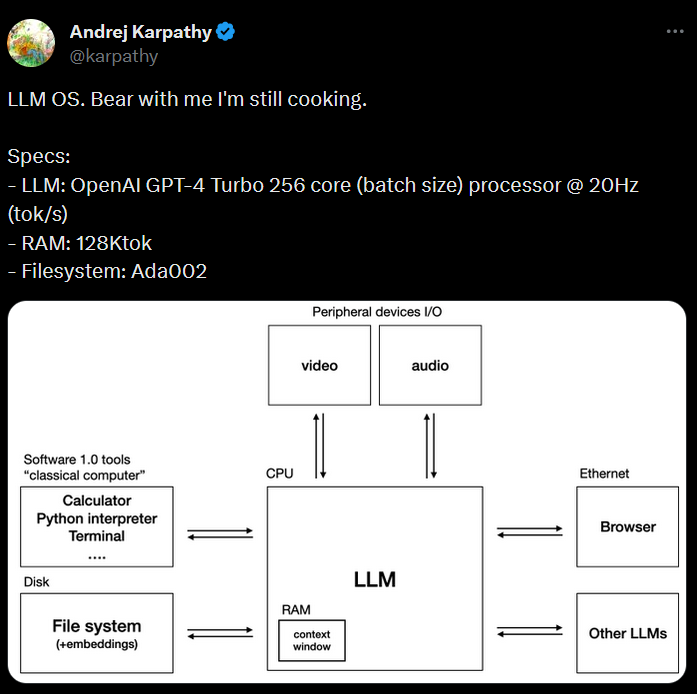
That is only the start. The concept of natively integrating LLMs and LAMs into gadgets is coming!
Keep up to date on this thrilling space of AI know-how by becoming a member of our AI e-newsletter and downloading our Python cheat sheets:
Technical Introduction to Giant Motion Fashions (LAMs)
LAMs embody a transformative strategy to human-computer interplay, shifting the paradigm from conventional graphical consumer interfaces to extra intuitive, action-oriented fashions.
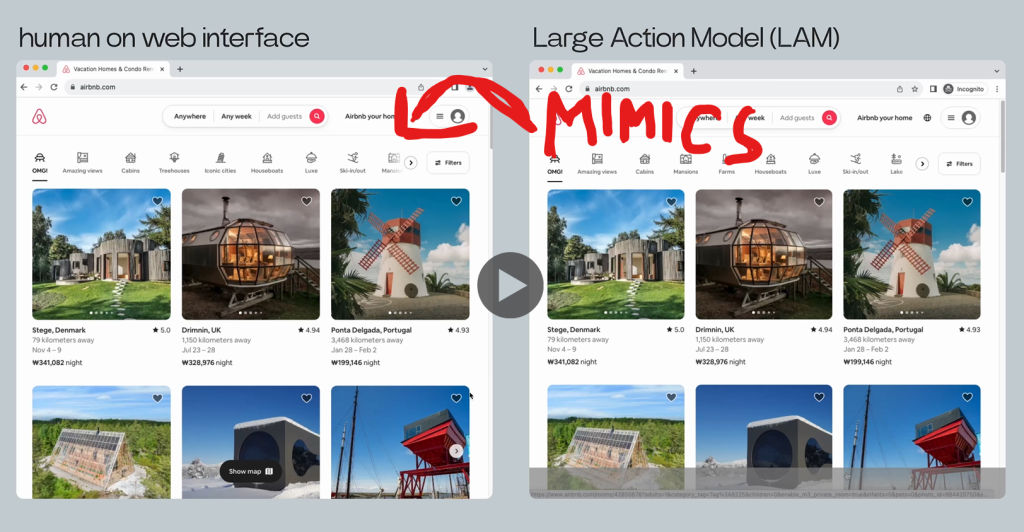
On the core of LAMs is the target to imitate and execute human actions inside laptop purposes, thereby making a extra pure and environment friendly consumer expertise.
In contrast to their predecessors, Giant Language Fashions (LLMs), which primarily give attention to understanding and producing textual content, LAMs are designed to know and replicate complicated consumer duties, starting from net navigation to application-specific operations. This functionality is achieved by way of a novel neuro-symbolic mannequin that blends the structured nature of symbolic algorithms with the adaptive studying prowess of neural networks.
The necessity for LAMs arises from the inherent limitations of standard AI fashions in comprehending and interacting with software interfaces. Normal language fashions, as an illustration, battle to suit the illustration of net purposes inside their contextual understanding as a result of verbose and noisy nature of those purposes. LAMs handle this problem by studying instantly from consumer interactions, bypassing the necessity for inflexible Software Programming Interfaces (APIs) and specializing in action-oriented studying.
An important side of LAMs is their capacity to study actions by way of demonstration, permitting them to adapt to variations in interfaces and execute duties reliably. This strategy not solely enhances the mannequin’s effectivity and accuracy but in addition ensures explainability and ease in job execution. By understanding the structural and logical composition of purposes, LAMs can carry out actions that align intently with human intentions, thus bridging the hole between customers and digital providers.
The mixing of LAMs extends to varied sensible domains, together with net navigation and automatic processes in cellular and desktop environments. The mannequin’s aggressive edge is clear in duties like net navigation, the place it demonstrates superior accuracy and latency in comparison with current strategies. This proficiency is underpinned by a technical stack that mixes transformer-style consideration, graph-based message passing, and program synthesizers guided by demonstrations and examples.
👉 “We consider that in the long term, LAM displays its personal model of “scaling legal guidelines,” [3] the place the actions it learns can generalize to purposes of every kind, even generative ones. Over time, LAM may grow to be more and more useful in fixing complicated issues spanning a number of apps that require skilled abilities to function.” — Rabbit Analysis
If you wish to study extra about scaling legal guidelines, take a look at our weblog article:
👉 AI Scaling Legal guidelines – A Quick Primer
Ceaselessly Requested Questions
What precisely is a Giant Motion Mannequin (LAM)?
A Giant Motion Mannequin (LAM) is a sophisticated AI system that may perform human duties on laptop purposes. In contrast to simply offering info or directions, LAMs can actively carry out duties like navigating web sites, filling out varieties, or on-line buying. They mix neural networks and symbolic reasoning to mannequin and execute numerous software duties.
Who created the primary LAM?
The primary LAM was developed by Rabbit Analysis Workforce, a pioneering AI firm. They launched Rabbit R1, a tool that makes use of LAM know-how to execute complicated duties on purposes utilizing pure language instructions.
How is LAM higher than different AI fashions?
LAMs have a number of key benefits:
- Accuracy: They carry out duties with excessive precision.
- Interpretability: LAMs can clarify their actions and logic in a transparent method.
- Pace: They full duties shortly by avoiding in depth knowledge processing or a number of inference steps.
- Simplicity: LAMs can deal with complicated duties with easy instructions and require minimal coaching.
Are you able to give some examples of what duties a LAM can carry out?
Certain! LAMs can carry out a spread of duties throughout completely different purposes:
- Flight Reserving: For example, reserving a flight on Kayak by specifying particulars like vacation spot, date, and price range.
- Kind Filling: Filling out varieties on Google Docs with required information and formatting.
- Grocery Purchasing: Purchasing on Instacart by including objects to the cart and trying out.
- Playlist Creation: Making a playlist on Spotify based mostly on style, temper, and artists.
- Content material Summarization: Producing summaries of Wikipedia articles by figuring out key factors and key phrases.
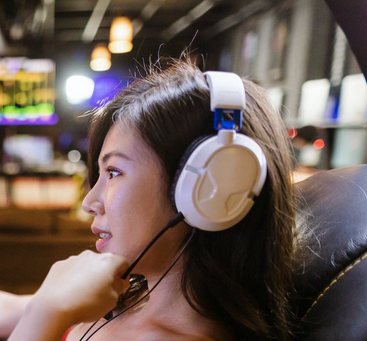
Emily Rosemary Collins is a tech fanatic with a robust background in laptop science, at all times staying up-to-date with the most recent developments and improvements. Aside from her love for know-how, Emily enjoys exploring the good outside, collaborating in area people occasions, and dedicating her free time to portray and pictures. Her pursuits and fervour for private progress make her an enticing conversationalist and a dependable supply of information within the ever-evolving world of know-how.
[ad_2]